Real-time Sampling, Estimation, and Inference in Networks
The Internet of Things (IoT) and social networks have emerged as powerful information platforms. But fundamental questions remain on how best to collect data and extract meaningful information. The data in these networks often comes from processes that evolve dynamically over time and space. For example, IoT networks involve numerous sensors monitoring time-varying physical processes, posing challenges in designing sampling and communication strategies for effective and timely estimation. Similarly, in tracking disease spread, such as COVID-19, efficient testing strategies are crucial for real-time monitoring and containment of outbreaks. Across these examples, the core challenge is to develop efficient, sequential sampling and testing methods that utilize real-time observations to enable accurate estimation and inference. My work in this domain is supported by an NSF CAREER award.
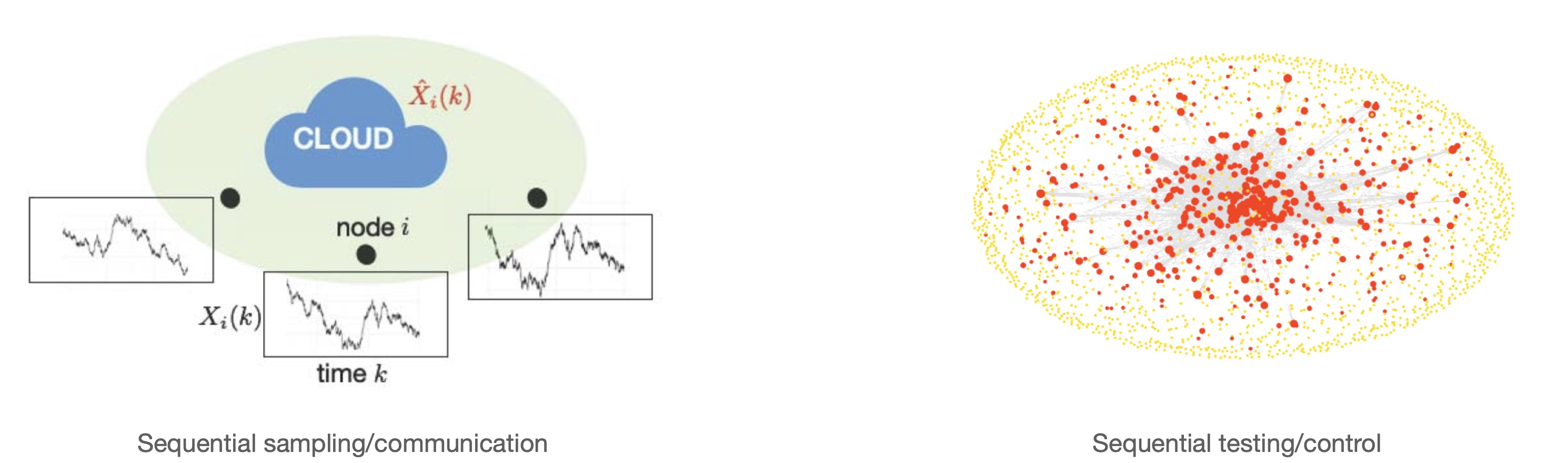
- Chen, Xingran, Konstantinos Gatsis, Hamed Hassani, and Shirin Saeedi Bidokhti. "Age of information in random access channels." IEEE Transactions on Information Theory 68, no. 10 (2022): 6548-6568.
- Chen, Xingran, Konstantinos Gatsis, Hamed Hassani, and Shirin Saeedi Bidokhti. "Age of Information in Random Access Channels." In 2020 IEEE International Symposium on Information Theory (ISIT), pp. 1770-1775. IEEE, 2020.
- Chen, Xingran, Xinyu Liao, and Shirin Saeedi Bidokhti. "Real-time sampling and estimation on random access channels: Age of information and beyond." In IEEE INFOCOM 2021-IEEE Conference on Computer Communications, pp. 1-10. IEEE, 2021.
- Chen, Xingran, and Shirin Saeedi Bidokhti. "Benefits of coding on age of information in broadcast networks." In 2019 IEEE Information Theory Workshop (ITW), pp. 1-5. IEEE, 2019.
- Chen, Xingran, Renpu Liu, Shaochong Wang, and Shirin Saeedi Bidokhti. "Timely broadcasting in erasure networks: Age-rate tradeoffs." In 2021 IEEE International Symposium on Information Theory (ISIT), pp. 3127-3132. IEEE, 2021.
- Bidokhti, Shirin Saeedi, and Aylin Yener. "On the Timeliness of Arithmetic Coding." In 2021 IEEE International Symposium on Information Theory (ISIT), pp. 2304-2309. IEEE, 2021.
- Chen, Xingran, Hesam Nikpey, Jungyeol Kim, Saswati Sarkar, and Shirin Saeedi Bidokhti. "Containing a spread through sequential learning: to exploit or to explore?." Transactions on Machine Learning Research.
- Kim, Jungyeol, Shirin Saeedi Bidokhti, and Saswati Sarkar. "Capturing COVID-19 spread and interplay with multi-hop contact tracing intervention." Plos one 18, no. 7 (2023): e0288394.
- Kim, Jungyeol, Xingran Chen, Hesam Nikpey, Harvey Rubin, Shirin Saeedi Bidokhti, and Saswati Sarkar. "Tracing and testing multiple generations of contacts to COVID-19 cases: cost–benefit trade-offs." Royal Society Open Science 9, no. 10 (2022): 211927.
- Arghal, Raghu, Eric Lei, and Shirin Saeedi Bidokhti. "Robust graph neural networks via probabilistic lipschitz constraints." In Learning for Dynamics and Control Conference, pp. 1073-1085. PMLR, 2022.
- Arghal, Raghu, Shirin Saeedi Bidokhti, and Saswati Sarkar. "Optimal Capacity-Constrained COVID-19 Vaccination for Heterogeneous Populations." In 2022 IEEE 61st Conference on Decision and Control (CDC), pp. 5620-5626. IEEE, 2022.
- Nikpey, Hesam, Saswati Sarkar, and Shirin Saeedi Bidokhti. "Group Testing with General Correlation Using Hypergraphs." In 2024 IEEE International Symposium on Information Theory (ISIT), pp. 3225-3230. IEEE, 2024.
- Nikpey, Hesam, Jungyeol Kim, Xingran Chen, Saswati Sarkar, and Shirin Saeedi Bidokhti. "Group testing with correlation under edge-faulty graphs." IEEE Transactions on Information Theory (2024).
- H. Nikpey, J. Kim, X. Chen, S. Sarkar and S. S. Bidokhti, "Group Testing with Correlation via Edge-Faulty Graphs," 2022 IEEE International Symposium on Information Theory (ISIT), Espoo, Finland, 2022, pp. 880-885, doi: 10.1109/ISIT50566.2022.9834777.
Minimizing age of information and estimation error for Markov processes
Timeliness of information transfer
Sequential testing and learning for disease processes
Group testing in networks (with correlation)
Learned Data Compression
Working with big data is impossible without data compression techniques that reduce the dimension and size of the data for storage and communication purposes and effectively denoise for efficient and accurate processing. In the past decade, learning-based compressors have shown great success in the task of compression using generative models. Their performance significantly surpasses traditional techniques such as JPEG (and variants) and their complexity is much lower than information-theoretically optimal solutions such as vector quantization (VQ) that have high complexity (exponential in dimension). Our aim is to investigate the fundamental limits of learning-based compressors and to develop novel learning-based compression methods that are informed by information-theoretic designs, with the goal of pushing performance towards the optimal.
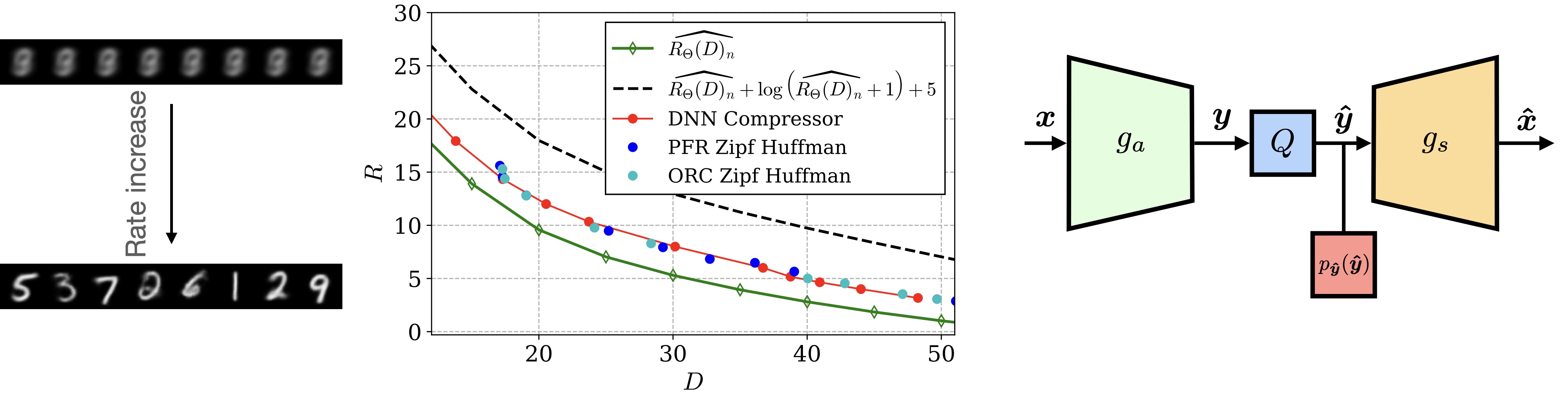
- Lei, Eric, Hamed Hassani, and Shirin Saeedi Bidokhti. "Neural estimation of the rate-distortion function with applications to operational source coding." IEEE Journal on Selected Areas in Information Theory 3, no. 4 (2022): 674-686.
- Lei, Eric, Hamed Hassani, and Shirin Saeedi Bidokhti. "Neural estimation of the rate-distortion function for massive datasets." In 2022 IEEE International Symposium on Information Theory (ISIT), pp. 608-613. IEEE, 2022.
- Lei, Eric, Hamed Hassani, and Shirin Saeedi Bidokhti. "On a Relation Between the Rate-Distortion Function and Optimal Transport." arXiv preprint arXiv:2307.00246 (2023).
- Lei, Eric, Hamed Hassani, and Shirin Saeedi Bidokhti. "Approaching Rate-Distortion Limits in Neural Compression with Lattice Transform Coding." arXiv preprint arXiv:2403.07320 (2024).
- Lei, Eric, Hamed Hassani, and Shirin Saeedi Bidokhti. "Neural Compression with Lattice Transform Coding." In International Zurich Seminar on Information and Communication (IZS 2024). Proceedings, pp. 14-14. ETH Zürich, 2024.
- Lei, Eric, Hamed Hassani, and Shirin Saeedi Bidokhti. "Out-of-distribution robustness in deep learning compression." arXiv preprint arXiv:2110.07007 (2021).
- Lei, Eric, Yiğit Berkay Uslu, Hamed Hassani, and Shirin Saeedi Bidokhti. "Text+ sketch: Image compression at ultra low rates." arXiv preprint arXiv:2307.01944 (2023).
Fundamental limits of learning-based compression
Neural Compression with information theoretic designs
Ultra-low rate regimes
Caching in Wireless Networks
Distributed edge caching has been one of the significant advancements in networking over the past decades. The concept involves replicating and storing content at the edge of the network, enabling faster local access while reducing the load on servers and the global network. Information-theoretic research has introduced novel coding frameworks for caching. My work focuses on edge caching in wireless broadcast networks, where I develop joint caching and coding strategies that yield substantial gains in both latency and throughput, scaling with the number of users. This research was partially supported by an NSF CRII award.
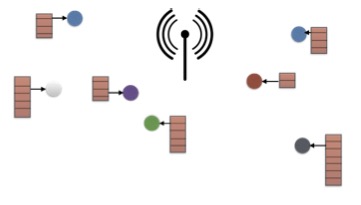
- Bidokhti, Shirin Saeedi, Michele Wigger, and Aylin Yener. "Benefits of cache assignment on degraded broadcast channels." IEEE Transactions on Information Theory 65, no. 11 (2019): 6999-7019.
- Wang, Chien-Yi, Shirin Saeedi Bidokhti, and Michele Wigger. "Improved converses and gap results for coded caching." IEEE Transactions on Information Theory 64, no. 11 (2018): 7051-7062.
- Bidokhti, Shirin Saeedi, Michele Wigger, and Roy Timo. "Noisy broadcast networks with receiver caching." IEEE Transactions on Information Theory 64, no. 11 (2018): 6996-7016.
- Timo, Roy, Shirin Saeedi Bidokhti, Michele Wigger, and Bernhard C. Geiger. "A rate-distortion approach to caching." IEEE transactions on information theory 64, no. 3 (2017): 1957-1976.
- Bidokhti, Shirin Saeedi, Michele Wigger, Aylin Yener, and Abbas El Gamal. "State-adaptive coded caching for symmetric broadcast channels." In 2017 51st Asilomar Conference on Signals, Systems, and Computers, pp. 646-650. IEEE, 2017.
- Heindlmaier, Michael, and Shirin Saeedi Bidokhti. "Capacity regions of two-receiver broadcast erasure channels with feedback and memory." IEEE Transactions on Information Theory 64, no. 7 (2018): 5042-5069.
Cloud Radio Access Networks (C-RANs)
In the next generation of 5G mobile networks, cloud radio access networks (C-RANs) are among the most promising technologies to attain a leap forward in spectral efficiency. This architecture was first proposed by IBM and China Mobile, and it combines advances in both wireless networks and cloud distributed processing. In traditional radio access networks, base stations are defined through a co-location of baseband units and radio heads. The radio heads transmit and receive the radio signals, and the baseband units process the signals before transmission and after reception. In a fundamental paradigm shift, C-RANs employ a large number of simple base stations that consist only of radio heads (RRUs), and aggregate the baseband units (BBUs) of multiple base stations together at central processors, connected to the RRUs through optical fiber or microwave links. The centralization of the BBUs allows for utilization of multiple RRUs for transmission to and reception from users to exploit diversity, joint information processing, coding, and design of transmission strategies across multiple RRUs to share spectrum. The vision of centralized processing and high density transmission promises spectral efficiency if challenges that thwart the large scale deployment of CRANs can be surmounted. We will realize this vision through a cross-layer investigation of C-RAN technology. This research was partially supported by NSF award #1850356 in collaboration between UPenn and Rutgers
- Dikshtein, Michael, Shirin Saeedi Bidokhti, and Shlomo Shamai Shitz. "Bounds on the capacity of the multiple access diamond channel with cooperating base-stations." In 2022 IEEE International Symposium on Information Theory (ISIT), pp. 3031-3036. IEEE, 2022.
- Saeedi Bidokhti, Shirin, Gerhard Kramer, and Shlomo Shamai. "Capacity bounds on the downlink of symmetric, multi-relay, single-receiver C-RAN networks." Entropy 19, no. 11 (2017): 610.
- Bidokhti, Shirin Saeedi, and Gerhard Kramer. "Capacity bounds for diamond networks with an orthogonal broadcast channel." IEEE Transactions on Information Theory 62, no. 12 (2016): 7103-7122.